Healthcare's Digital Transformation: Data Strategy Issues
Last Updated:
March 21, 2024
March 12, 2024
Introduction
There is no doubt about the transformative potential of big data and analytics. This is particularly true for the Life Science sector as implementing technologies and ideologies can revolutionize drug development, tailor medicines to individual needs, dramatically improve patient care and more. The data supports this, with the longest running report of Fortune 1000 CIOs by Wavestone, showing 87.9% of companies believe “investments in Data & Analytics are a Top Organizational Priority.”
Such great promise and high buy in should mean easy cultural adoption, right? Well not exactly. The 2024 report shows there has been a notable improvement; the percentage of top executives facing significant challenges in culture, people, and process/organization decreased from a staggering 90% in 2020 to 77.6% in 2024. While this reduction signifies a positive trend towards addressing these issues, the fact that more than three-quarters of leaders still encounter these problems underscores the widespread nature of these challenges. The persistently high percentage highlights the need for continued and focused efforts to overcome these barriers.
In an effort to help further lower the 77.6%, this article aims to cover the benefits of data in the Life Science sector, highlight common culture issues, and provide some solutions to the problem. If your organization is among those facing these struggles, know that you are not alone.
Benefit of data in Life Science sector
The life science sector stands on the brink of a digital transformation, powered by the strategic use of data. It's clear that its impact is far-reaching, transforming every facet from research and development to patient care and beyond. Below are some examples of what this data-driven culture can improve.
- Accelerating Drug Development: One of the most significant benefits of data lies in its ability to streamline and accelerate the drug development process. By harnessing big data and advanced analytics, life science organizations can identify potential drug candidates faster than ever before. This not only reduces the time and cost associated with bringing new treatments to market but also allows for more targeted and effective therapies.
- Enhancing Personalized Medicine: Personalized medicine, tailored to the individual characteristics of each patient, is becoming a reality thanks to data. By analyzing vast datasets, including genetic information, researchers can understand how different people are likely to respond to treatments. This leads to more effective and personalized care plans, improving patient outcomes and reducing the likelihood of adverse reactions.
- Improving Clinical Trials: Data analytics revolutionize the way clinical trials are conducted. By leveraging data, scientists can design better trials, select suitable candidates more accurately, and monitor results in real time. This not only increases the efficiency and efficacy of trials but also enhances patient safety by identifying potential issues earlier in the process.
- Driving Operational Efficiency: Beyond research and patient care, data also plays a crucial role in enhancing the operational efficiency of life science organizations. From streamlining supply chains to optimizing resource allocation, data-driven insights help companies operate more efficiently, reduce costs, and respond more quickly to market changes.
- Fostering Innovation: At its core, the utilization of data fosters an environment of innovation within the life science sector. By providing a wealth of information and insights, data encourages researchers to explore new hypotheses, uncover hidden patterns, and push the boundaries of what's possible in medical science.
- Informing Policy and Decision-Making: Lastly, data aids in the development of more informed policies and decisions. By analyzing trends, outcomes, and costs, policymakers and healthcare providers can make evidence-based decisions that lead to better health outcomes and more efficient use of resources.
Digital transformation in healthcare - Key challenges
As we identified earlier in this article, culture, people, and process/organization challenges are the biggest obstacles for companies to achieve digital transformation and become data-driven. Identifying specific challenges is key to developing a solution.
- Resistance to Change: Cultural norms and resistance to change within organizations can impede the adoption of new data strategies, especially when there is a lack of buy-in from key stakeholders. This can be especially present in the Life Science sector which tends to lean risk averse.
- Overemphasis on Buzzwords and End Products: Initiatives often start with buzzwords like 'Gen AI', focusing primarily on the final product without addressing underlying flawed infrastructures and processes. This inclination towards technology is because technology is tangible, you can talk to vendors and see their websites, however, consistent findings from the annual report reveal that technical challenges constitute only 23.4% of the issues; the bulk stems from ingrained cultural problems within organizations. Meaning, adopting new technologies without addressing these foundational processes is likely to lead to suboptimal outcomes.
- Lack of Alignment: When different departments or teams pursue the same objective without proper coordination, it results in alignment issues. These misalignments act as barriers to the seamless execution of unified data strategies, causing data management inefficiencies. Often, this situation stems from a lack of centralized guidance and strategic direction. It is important for organizational leaders to bridge these gaps through a top-down approach, ensuring all components work in harmony towards the collective aim.
- Cultural Complexity: Large enterprises especially face this issue. Cultural complexities, such as diverse social and cultural preferences, values, and disparities in knowledge, can pose challenges in implementing data strategies, particularly in institutions spanning developed and developing countries. This is why taking the time to align fundamentally is especially important for the enterprise.
- Misplaced Prioritization of Data: Often, data is viewed as a technology concern for IT to handle, while other teams focus on deliverables like patient service or clinical trials. This perspective is problematic as data is crucial for analyzing and ensuring the success of these initiatives. Typically, data management is considered too late in the process, when the necessary data may not have been effectively captured for analysis.
Solutions to digital transformation challenges
Questioning the status quo
Pharma companies tend to be risk averse due to the nature of the data they are responsible for. This leads to limitations and constraints to innovative solutions. These constraints are often accepted without understanding the rationale behind these constraints or challenging them. New technology offers new ways to manage data that were not available in the past. If a company remains the most risk averse and the most conservative this will stifle innovation. The key takeaway is that it is not only about technology but about the new process potential that this new tech provides.
Focus on fundamentals
This goes back to the foundation. Technology changes, initiatives change, but data truths do not. These truths involve thinking about end-to-end processes, data quality, documentation, good guidelines conventions, data governance, reducing points of failure ect. It is easy to get swept up by the latest trend and want to jump in, but you cannot build a house on quicksand; new trends like Gen AI still require these things underneath.
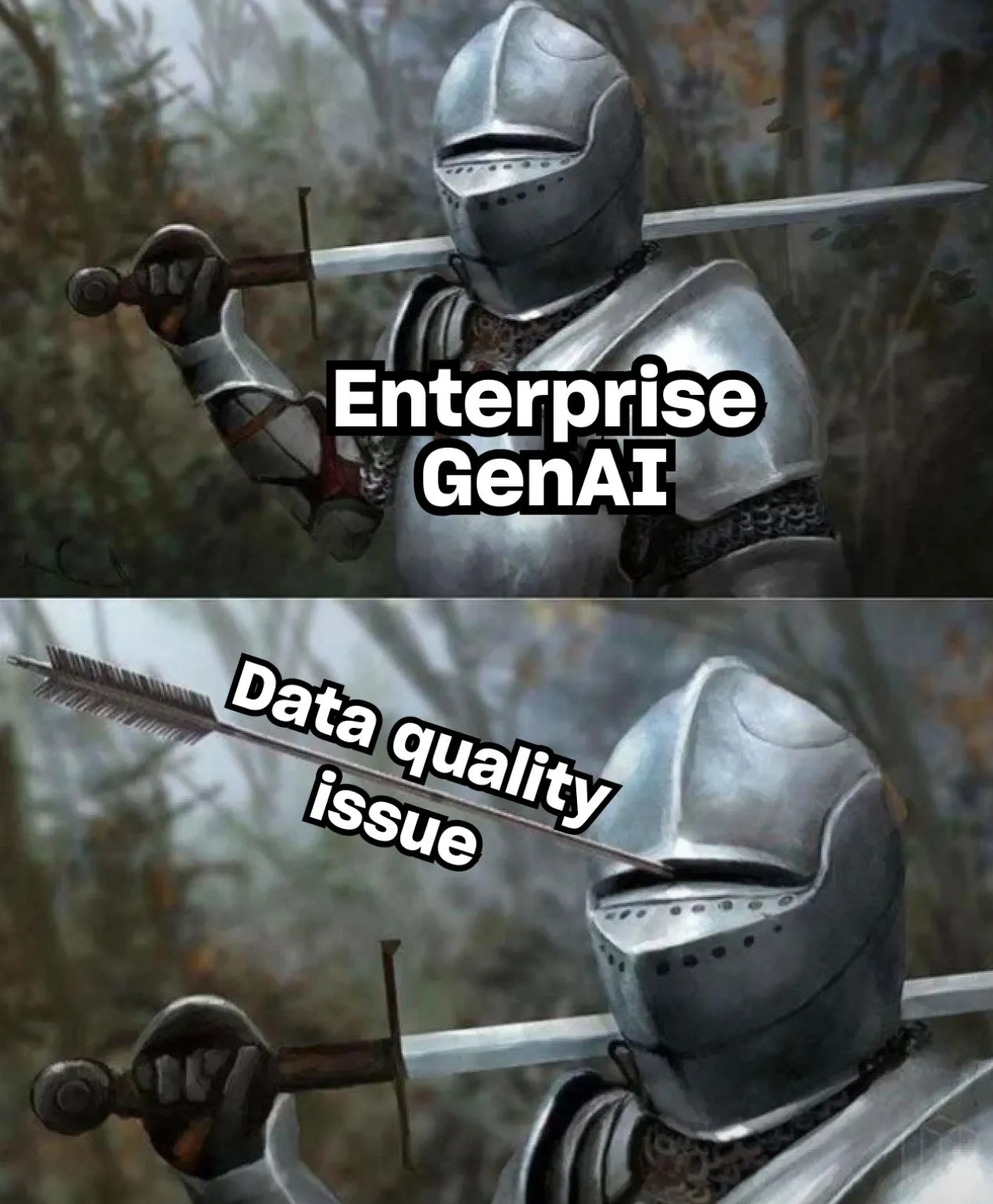
Fundamental alignment
This starts with aligning the team. Alignment is one of the 3 core pillars to a data-driven culture. People will start out thinking they are on the same page because they are using the same terminology. But we all have different ideas influenced by our experiences. So, it is important to gather all stakeholders and go through a true alignment process which includes figuring out the current state, pain points, and aligning on the solution.
Fundamental alignment includes adopting a top-down approach. The efforts one team is making are sure to affect the efforts of another. Leadership must align and connect the dots between all moving parts and understand that things are not living in isolation. This will solve headaches downstream and ensure a smoother process.
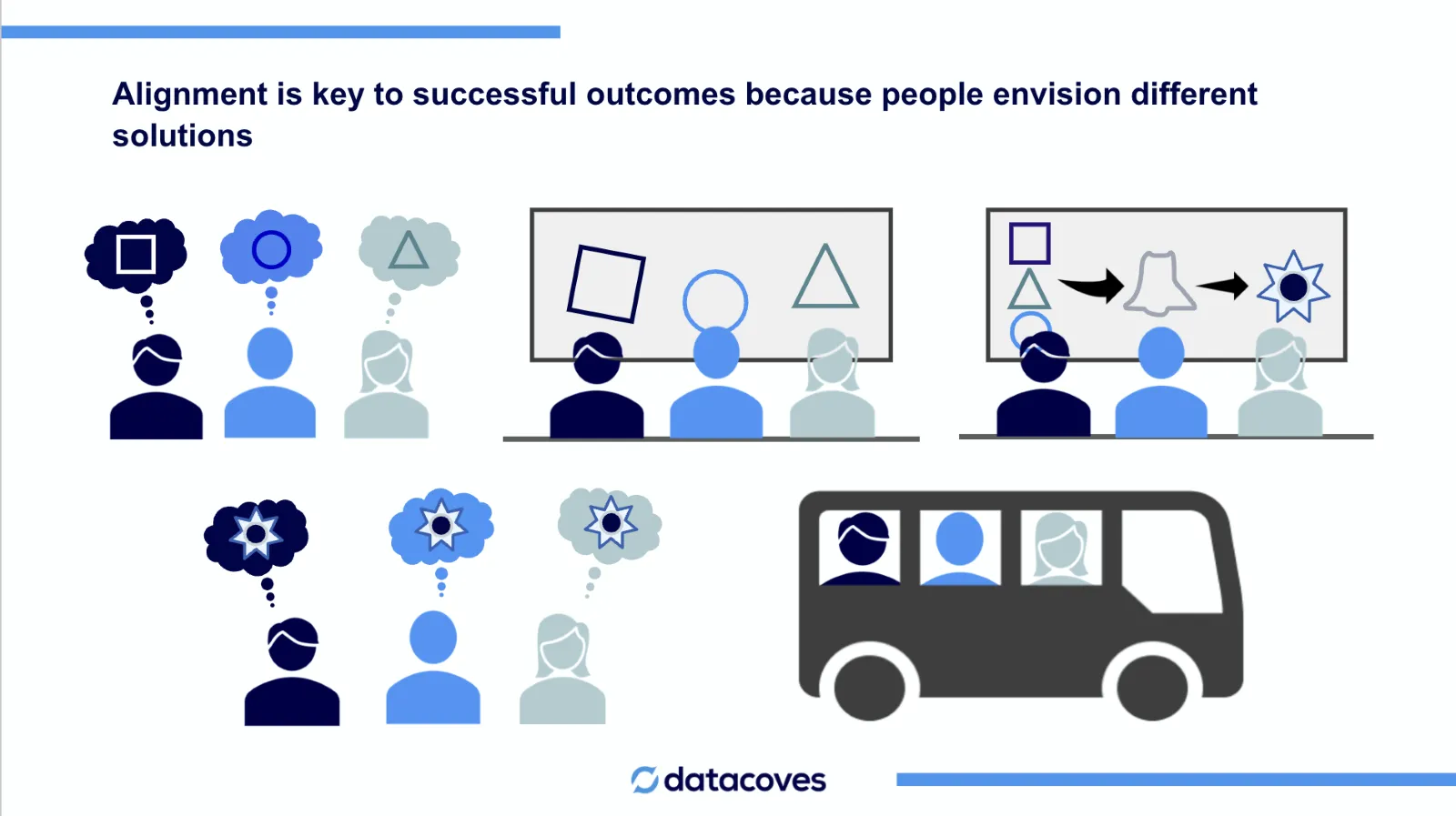
Quick wins
Alignment alone won’t be enough to combat culture challenges. True alignment can lead to overly ambitious projects that may prove difficult to execute. This is the classic trap of trying to do too much at once. It is important to start with manageable, small-scale projects that can provide immediate benefits. These quick wins are vital for positively influencing the organizational culture. Additionally, they help maintain momentum: if a larger initiative is slow to yield results, these smaller successes ensure continuous progress. By allowing for ongoing adjustments and reprioritizations, these projects help prevent initiatives from being abandoned.
Conclusion
While the potential of big data and analytics in transforming the Life Science sector is undeniable, and investment in data is on the rise, the journey towards a data-driven culture remains one of the biggest challenges for data integration. The path forward requires a balanced approach that combines technology with fundamental changes in culture and processes. By embracing these changes, the life sciences sector can overcome existing barriers and fully harness the power of data to advance medical science and improve patient outcomes. At Datacoves we are passionate about helping companies achieve a data-driven culture. See how Datacoves helped Johnson&Johnson innovate their tech stack.
Prefer a Video?
This article was inspired by RAN BioLinks’ podcast episode “Why Life Science Organizations Fail to Implement Effective Data Strategies”. The detailed conversation with Noel Gomez, a seasoned expert in data management within the life science industry, explores the critical challenges and innovative solutions for effective data strategies in healthcare.