Companies are investing heavily to become data-driven and to democratize data access. However, many are not achieving the transformative outcomes they expected.
The core issue? A lack of trust.
This mistrust stems from a lack of focus on core aspects that ensure a robust data-driven culture and critical mistakes in these areas.
Fortunately, these mistakes are self-inflicted which means they can be fixed, and this article aims to help highlight and address these pitfalls. By understanding and adhering to the core pillars of a data-driven culture and avoiding the common mistakes, organizations can develop and maintain a data-driven culture that people can trust.
What is data-driven culture?
It is no secret that there is power and opportunity in data, and data-driven culture is the approach which aims to take advantage of that.
A data-driven culture is not about hastily adopting the latest tools or technologies in the hope of resolving data challenges. This common mistake often leads to a focus on immediate results or 'shiny objects', such as acquiring cutting-edge technology or hiring new talent. Unfortunately, this approach tends to overlook essential priorities and gradually erodes the foundation of a data-driven culture: Trust in the data.
Many companies struggle with effectively using analytics because they overemphasize these immediate goals – the 'destination' – rather than appreciating the foundational journey necessary for impactful analytics. This journey involves more than just technology; it requires a shift in mindset and approach.
Data-driven culture represents an organizational approach where data is the cornerstone of decision-making processes. In such a culture, decisions are primarily informed by data analysis, rather than relying exclusively on intuition or past experiences. This approach involves strategically employing data at every level of the organization. It fosters an environment where data is not just an asset but the main driver of strategy, innovation, and operational choices. By harnessing the power and opportunities offered by data, a data-driven culture ensures that decisions across the organization are grounded in solid evidence and analytical insight, enhancing the overall decision-making quality and efficacy.
Key features of data-driven culture include:
Empowered Decision Making: Decisions are based on data analysis, leading to objective and impactful outcomes.
Accessibility of Data: Data is accessible across the organization, breaking down silos and empowering all employees.
Investment in Technology: Adequate tools and technologies are provided for effective data collection and analysis.
Data Literacy: Continuous training is provided to enhance the workforce's understanding and use of data.
Quality and Governance: High standards of data accuracy and security are maintained.
Agility: The organization adapts quickly to insights derived from data.
Collaborative Integration: Data insights are shared and integrated across various functions.
Outcome-Focused: Emphasis on measurable results driven by data insights.

Building a data-driven culture: Core pillars
All of that sounds great, but how do we achieve a data-driven culture?
Like mentioned earlier in the article, true success in analytics comes not from merely chasing new tools or methodologies but from establishing three core pillars as part of a Data-Driven Culture:
- Fundamental Alignment: It's essential to align analytics strategies with core business objectives, ensuring everyone involved shares a common vision and understanding.
- User-Focused Solutions: The end goal of analytics should be to serve the user's needs. This involves designing solutions that are practical, add real value, and enhance the decision-making process.
- Efficient Data Management: Implementing robust data processes is key. This involves ensuring data accuracy, accessibility, and understandability, which are crucial for informed decision-making.
By refocusing on these foundational elements, businesses can drive more meaningful and sustainable results from their analytic endeavors, leading to overall project success and satisfaction.
Let's dive deeper into the core pillars and examine the common pitfalls within each pillar that I have observed lead to challenges.
Lack of alignment reduces faith in the solution: Fundamental alignment
Fundamental alignment is about synchronizing analytics strategies with the organization's core business objectives. This ensures everyone involved, from executives to frontline employees, share a common vision and understanding of what analytics aims to achieve. This alignment is crucial for creating a unified direction in data-driven initiatives and ensuring that every analytics effort contributes meaningfully to the overall business strategy.
This sounds great right? So much so that every project I've participated in began with high hopes and enthusiasm. Initially, there was a sense of unity – funding secured, partnerships with vendors established, and the latest technology acquired. This honeymoon phase of the data driven transformation, filled with optimism, had everyone working diligently, with management receiving regular updates and a general belief that we were on the right track.
Pitfall
The real test emerged when critical decisions were required. This was the point where the honeymoon phase often faded, revealing a lack of true alignment. Meetings became prolonged discussions where the team struggled to reach consensus. This challenge stemmed from either not spending enough time initially to ensure everyone was on the same page or not conducting a discovery phase at the start of the project.
Although we agreed on high-level objectives like digital transformation and self-service analytics, there was a misalignment in our deeper understanding and perspectives. We were each influenced by our varied backgrounds and expertise in different aspects of the project.
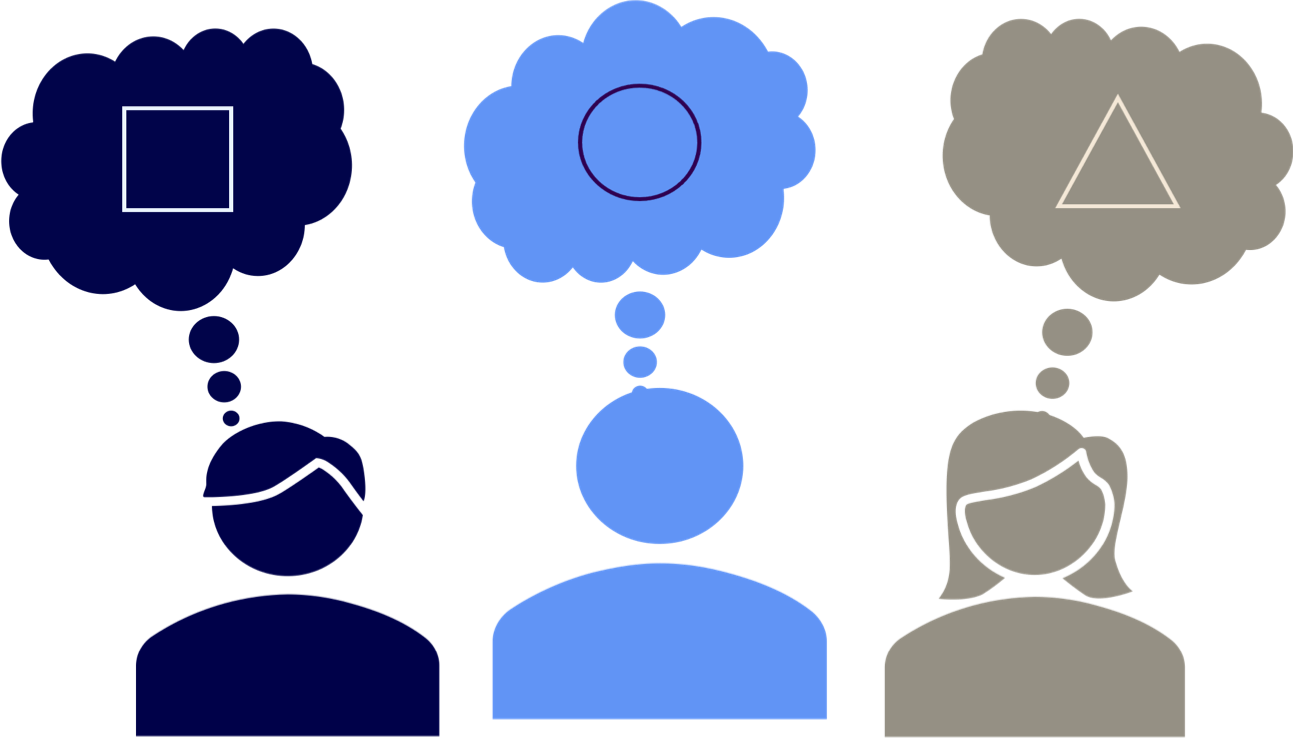
Solution
This led me to a crucial fact: the importance of alignment before action. In projects where we dedicated time upfront for structured alignment and thorough product discovery, we not only achieved better estimations but also greater overall satisfaction. It became evident that successful analytics projects require a deep understanding of business objectives, the current state, potential risks, and a clear prioritization of features. This was because we developed a clear understanding and set up expectations that people could rely on throughout the course of implementation.
Crucially, alignment also involves clarity on what the project will not address, alongside the criteria for prioritization such as quality, completeness of features, and usability. Embracing agility does not mean forgoing thorough planning.
Ultimately, building trust in any project begins with listening, creating a shared vision, and setting the right expectations from the start. A well-defined and achievable plan, understood and agreed upon by all, is the foundation of success.
Bad user experiences erode confidence: User-focused solutions
The end goal of analytics should be to serve the user's needs and involve designing practical solutions that add real value and enhance decision-making processes. This means creating analytics tools and processes that are intuitively aligned with how users work and make decisions, ensuring that these tools are not just technically proficient but also practically useful.
Pitfall
There are two pitfalls to avoid.
1. Trying to please everyone often leads to pleasing no one.
This is a common scenario in many companies where technical teams strive to meet all demands. Despite their efforts to deliver on projects, dissatisfaction with the end results is frequent.
2. Not addressing the actual user pain points.
This happens when the user does not actually get a good working solution out of the process.
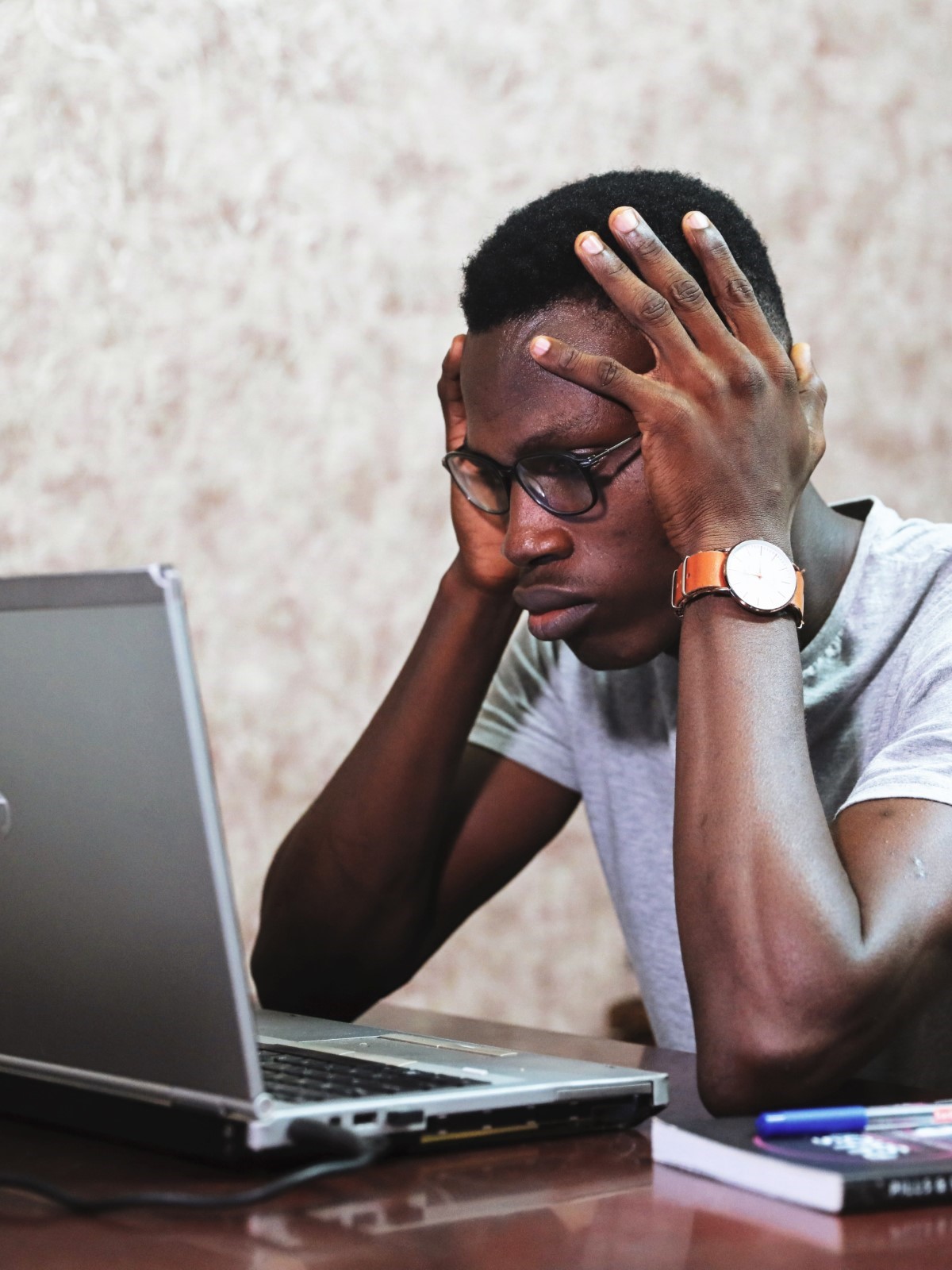
Solution
During discovery it is important to discuss what is in scope, out of scope, essential, and nice to have. By categorizing this way you can better understand the needs of the group and use it to guide the process. With this process done, you can move forward with confidence that you are addressing the most important pain points.
Now that we have defined the pain points, the next step is to fully understand. The key is to not only understand their needs but the reasons behind them. What are the goals they're trying to achieve? What are the shortcomings of their current methods? Is the new process or tool genuinely an improvement over what they currently have? For example, if users need to navigate a tool quickly, finding ways to reduce unnecessary clicks and simplifying access becomes important. Sometimes, it's more practical to keep an existing process unchanged until other parts are enhanced. By bringing the most critical information to the forefront, the solution becomes more user centric.
It is important to have these needs in mind at the beginning of the project and strive to truly understand. If not, you risk investing time, money, and resources in a tool that users don't need, and this can have a detrimental effect on the overall culture.
More importantly, this approach allows you to justify your decisions and explain why certain aspects are prioritized over others. When users see that their needs and challenges are understood and addressed, they are more likely to trust and accept the solutions provided. This trust is built through consistently demonstrating that their best interests are at heart.
Data driven transformation: Efficient data management
Efficient data management involves implementing robust processes to ensure data accuracy, accessibility, and understandability. This pillar is key to informed decision-making as it underpins the reliability of data-driven insights. Effective data management includes organizing, storing, and safeguarding data to make it readily available and useful for users across the organization.
Pitfall
Let's face it, your data processes get no love. This is usually because they are "too technical." Users often do not concern themselves with databases, schemas, tables, or columns, let alone the process that turns raw facts into business-ready insights. It is easy for management to get excited about a fancy dashboard and the potential of Machine Learning and Gen AI, but when it comes to the actual data, interest tends to wane.
It makes sense; most people don't understand how the power grid works. We take it for granted that we flip a switch and expect the lights to turn on. We move on without a second thought. No one really cares about electricity until something goes wrong. Similarly, in many organizations, data issues often go unnoticed until a failure occurs. Sometimes these issues are immediately apparent, but other times they are silent. When a failure does happen, there is a scramble to fix it. Meetings are held, issues are identified, and patches are implemented to "prevent" future failures. However, the best time to think about potential problems isn't after they happen, but before — building systems that anticipate and are designed for resilience.

The real issue is that the process from raw data to insights isn't often viewed as a single system. It is all interconnected and should be treated as such. In the world of analytics, it sometimes feels like companies are trying to build a mansion on a foundation of quicksand. Initially, everything seems fine, and everyone is busy with their tasks, but when the foundation starts to give way, the focus shifts to propping up the weak points. You can't effectively build on quicksand; you need solid, repeatable processes from the start.
Solution
The focus should be on building systems that anticipate challenges and are designed for resilience. This involves integrating data management practices into the company's culture from the start, ensuring users trust the data and the processes that generate insights. If you want effective collaboration and impact analysis, these are difficult to retrofit later — they need to be part of the initial plan. Documented analytics isn't a magical solution; it needs to be ingrained in the culture and process from the beginning. The good news is that there are many examples and best practices from those who have navigated these challenges successfully.
For users to truly trust in analytics, they need to have faith in the data and the processes that generate it. They need to see and believe in a robust system built on a solid foundation.
Conclusion
To achieve a data-driven culture, companies must refocus on three core pillars: fundamental alignment, user-focused solutions, efficient data management, and avoid common mistakes in these areas. Success in analytics isn't about chasing new tools or methodologies but about building a robust system from the ground up, aligning everyone's vision, and creating practical, value-added solutions. Prioritizing foundational elements over immediate shiny objects will lead to more meaningful, sustainable results and will build trust in the analytics process.